Understanding Data Annotation in Machine Learning for Home Services
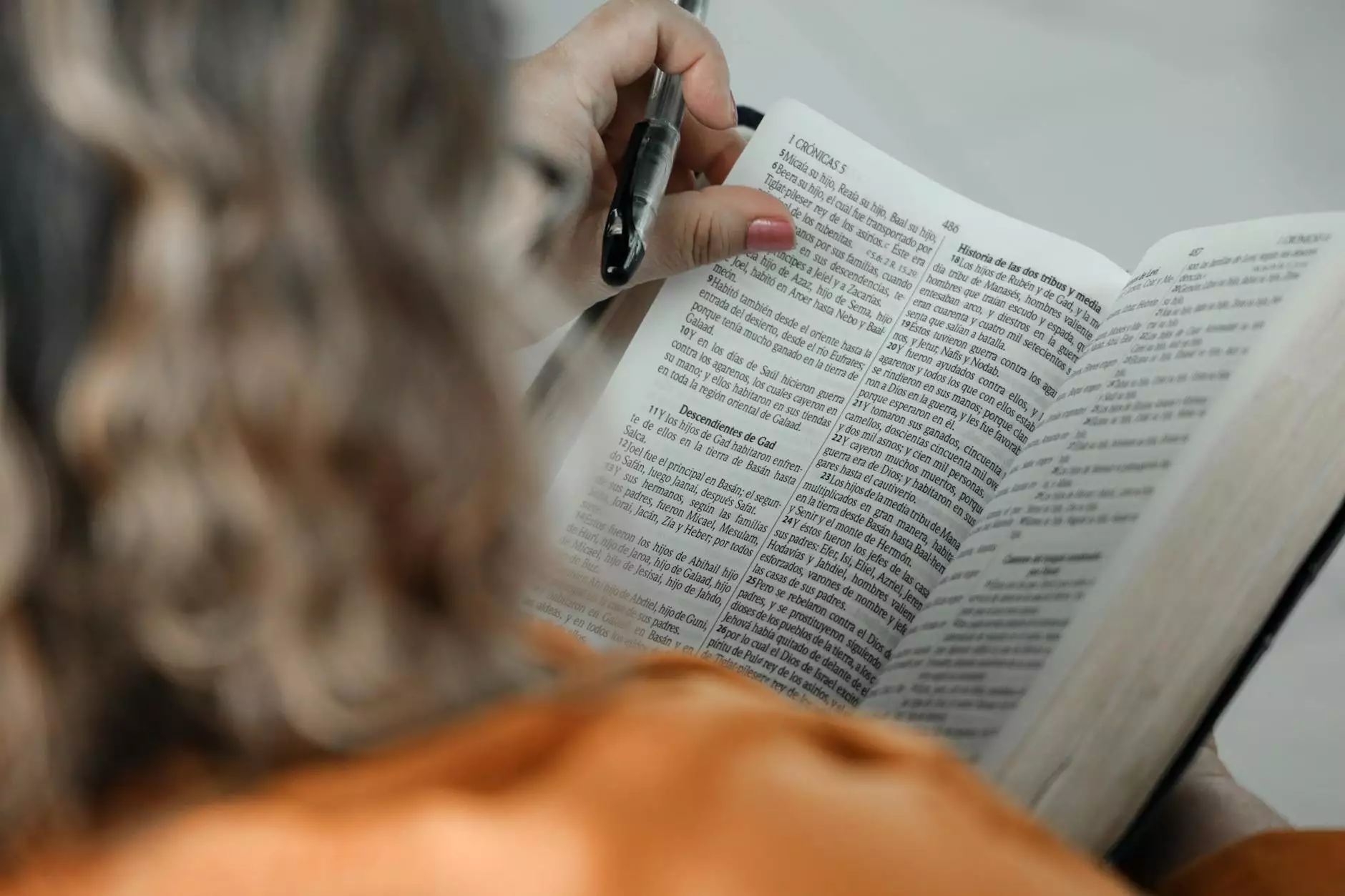
Data annotation is a crucial step in the machine learning process, especially when it comes to enhancing various industries, including home services like locksmiths and key cutting. In this article, we will delve into the manifold aspects of data annotation, its significance in machine learning, and how it can tremendously boost the efficiency and effectiveness of businesses in the home services sector.
The Basics of Data Annotation
Data annotation is the process of labeling or tagging data so that it can be used for machine learning models. This process involves various types of data, including text, images, and audio files. By providing context and meaning to raw data, annotation enables machine learning algorithms to learn and make predictions.
Types of Data Annotation
There are several types of data annotation, each tailored to suit different forms of data:
- Image Annotation: Involves labeling images with relevant tags or bounding boxes to help models recognize different objects.
- Text Annotation: Entails annotating text for sentiment analysis, entity recognition, and other linguistic applications.
- Audio Annotation: Involves tagging audio files, often for speech recognition or identifying sounds.
Why Data Annotation is Vital for Machine Learning
Data annotation is fundamental in the development of accurate and reliable machine learning models. Here are several reasons why it is essential:
1. Enhances Model Accuracy
Without precise data annotation, machine learning models may struggle to make accurate predictions. Quality annotations help the model to learn and generalize better. For example, if a machine learning model is trained in the home services domain, annotated images of different types of locks can improve its ability to identify locks based on various characteristics.
2. Provides Structured Data
Unstructured data is often difficult to analyze. Data annotation transforms unstructured data into structured formats, making it easier for machine learning algorithms to process and learn from it.
3. Enables Different Applications
Different forms of data can lead to varied applications. For instance, text annotations can be used to improve customer service chatbots in the locksmith industry by helping them interpret customer queries and provide accurate responses.
Data Annotation in Machine Learning: Applications in Home Services
Home services, particularly those concerning keys and locksmithing, can greatly benefit from the implementation of data annotation in machine learning. Below, we will explore some pertinent applications.
1. Automating Customer Support
Chatbots equipped with machine learning capabilities can enhance customer interactions. By using annotated text data from prior interactions, these bots can understand customer issues, provide relevant solutions, and improve over time as they learn from new data.
2. Optimizing Inventory Management
Machine learning algorithms can analyze annotated data to optimize inventory levels in locksmith businesses. For instance, understanding which keys are requested most frequently can help businesses stock them better and reduce wait times for customers.
3. Predictive Maintenance of Tools
Annotated data on tool performance can enable machine learning models to predict when maintenance is needed, thus preventing potential failures and ensuring that locksmiths have the tools they need to operate effectively.
The Role of Quality in Data Annotation
The quality of annotated data can significantly affect the performance of machine learning models. High-quality data annotation involves accuracy, consistency, and relevance. Below are key factors that contribute to high-quality annotations:
- Accuracy: It is essential that annotations precisely reflect the content. For instance, mislabeling an image of a lock can lead to faulty model predictions.
- Consistency: Annotations must be consistent across the dataset to reduce confusion. A consistent labeling scheme should be applied throughout.
- Relevance: Annotations should directly relate to the tasks that the machine learning model is designed to perform, ensuring that the data is useful for its intended purpose.
Challenges in Data Annotation
While the benefits of data annotation are plentiful, several challenges can arise:
1. Time-Consuming Process
Data annotation can be extremely time-consuming, especially for large datasets. Businesses need to allocate sufficient resources, both human and technological, to ensure that the annotation is done correctly and efficiently.
2. Subjectivity
Some types of data annotation can be subjective. For instance, different annotators may interpret the same data differently, leading to inconsistencies. Establishing clear guidelines and training for annotators can help alleviate this issue.
3. Scalability
As businesses grow, the volume of data requiring annotation can increase significantly. Developing scalable annotation processes or tools can be critical for handling large datasets efficiently.
Best Practices for Effective Data Annotation
To ensure that data annotation successfully contributes to effective machine learning outcomes, businesses should employ several best practices:
- Define Clear Objectives: Before commencing data annotation, it's vital to outline the objectives clearly. Understanding the end goal will guide annotators in making informed decisions.
- Use the Right Tools: Leverage technology to aid the annotation process. Tools that facilitate collaboration and real-time feedback can greatly enhance productivity.
- Engage Skilled Annotators: Employ annotators with domain knowledge and experience. This is particularly crucial in specialized fields such as locksmithing, where industry knowledge can lead to more accurate annotations.
- Regular Quality Checks: Implement quality assurance processes to regularly review annotations and ensure they meet set standards.
Conclusion: The Future of Data Annotation in Home Services
As the home services industry increasingly incorporates technology and data-driven solutions, the relevance and importance of data annotation in machine learning will continue to rise. The ability to efficiently manage and interpret data can empower businesses like those in keys and locksmithing to enhance their services, improve customer interactions, and optimize operations.
Keymakr.com stands at the forefront of this evolution, offering innovative solutions that harness the power of machine learning and data annotation to deliver exceptional services in the locksmithing domain. Embracing these technologies not only positions businesses as leaders in their field but also paves the way for continuous improvement and satisfaction in home services.
For more information on how data annotation and machine learning can transform your home service business, visit Keymakr.
data annotation machine learning